In the ever-evolving landscape of artificial intelligence, one term continues to captivate the minds of AI enthusiasts – Deep Learning (DL). Beyond its meaning as a buzzword, deep learning represents the cutting edge of AI, enabling machines to learn from vast datasets and make decisions in a way that mirrors human thought processes. But what lies beneath the surface of this technology, and how does it shape our present and future?
It profoundly impacts our technological world, from revolutionizing industries to enhancing everyday experiences. This powerful AI paradigm ushers in a new era of innovation and disrupts traditional methodologies. It also means endless possibilities in a place where knowledge meets creativity.
Keep reading as we touch on neural networks and decipher the inner workings of deep learning models.
Understanding deep learning
DL models are a byproduct of machine learning and artificial intelligence (AI) that can mimic how humans develop knowledge. It’s built upon layers of AI technology, where each layer processes data in a hierarchical manner, allowing the system to learn complex ideas. This layered approach eliminates confusion by enabling the system to automatically discover the features needed for pattern recognition.
Deep learning is valuable because it has the potential to transform countless industries by:
- Automating Tasks
- Making Predictions
- Uncovering Insights
The datasets in question would require more work for humans to process efficiently. Its ability to handle complex and unstructured data makes it a powerful tool for solving problems across domains.
Deep learning AI examples
Deep learning AI examples are everywhere and are integral to people’s daily lives, changing many industries and driving technology forward. Remember, DL models come from layers of neural networks. Each layer takes insight from the previous layer to sharpen its accuracy and improve its prediction capacity.
Deep learning neural networks are one of the closest attempts of technology to mimic the human brain. It uses an evolving toolset of data inputs, weights, and bias. These elements collaborate to accurately identify, categorize, and describe objects.
Here are some deep learning AI examples we use in everyday life:
- Natural Language Processing: DL models analyze and interpret human language, enabling sentiment analysis, language generation, and text summarization.
- Text-to-Speech Tools: TTS tools are SaaS that convert written text into spoken words. These tools allow users to listen to written content instead of reading it. We use TTS tools content-on-the-go and as accessibility features for people with visual impairments or language learning difficulties.
- AI Voices: Synthesized voices created by AI technology. These voices use DL algorithms and sound natural and human-like. We utilize AI voices in virtual assistants, navigation, and accessibility tools.
- Language Translations: DL algorithms run language translation services like Google Translate to remove communication barriers in a globalized world.
Deep learning models can recognize new features in the data they’re working with, but why would this be important? It removes lengthy time obligations while training deep-learning neural networks. This capability allows algorithms to adapt and improve their predictions without needing manual adjustments.
Because of this automation, deep learning models can continuously enhance their accuracy and performance, making them highly valuable.
Deep learning vs. machine learning
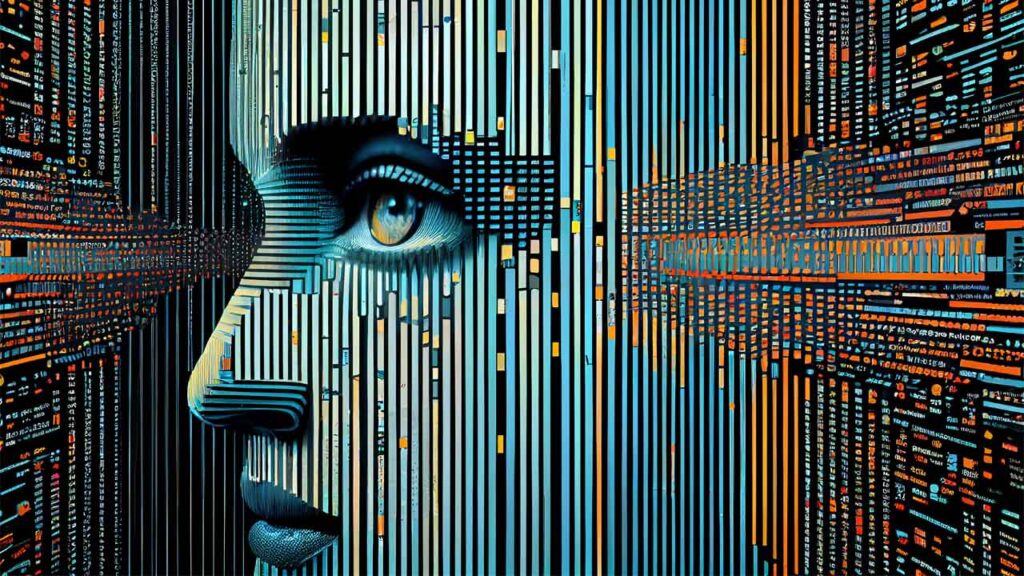
When people think of deep learning vs. machine learning, they don’t always realize that both are sub-categories of AI. Let’s take that one step further, as deep learning is only possible because of machine learning. The main difference comes from how they handle data and learn from it.
ML relies on structured, labeled data to make its predictions. The algorithm requires structured data organized into tables with defined features. With this structured data, ML models can improve with experience. DL sets itself apart because it can work with unstructured data, like text and images, without pre-processing.
It automates the feature extraction process, reducing the reliance on human experts. To illustrate the difference, consider the task of recognizing a cat in an image. In machine learning, a human might need to manually identify and define cat features, like ears, whiskers, and tail, in the image before the algorithm can make predictions.
In deep learning, the algorithm can learn to recognize these features independently, eliminating the need for human intervention.
Understanding the distinction: Machine learning vs. AI
When discussing machine learning vs AI, it’s essential to recognize that machine learning is simply a complex tool AI uses to mimic human behaviors. Put another way, AI refers to the broader concept of machines carrying out tasks in a way we would consider “smart.” ML enables systems to learn and improve from experience automatically.
In essence, AI encompasses the overarching goal of creating intelligent machines, while machine learning is a powerful tool to achieve that goal. Therefore, while AI is a crucial component of machine learning models, it’s the capacity of ML that allows these intelligent applications to accomplish so much.
Does artificial intelligence compare to human intelligence?
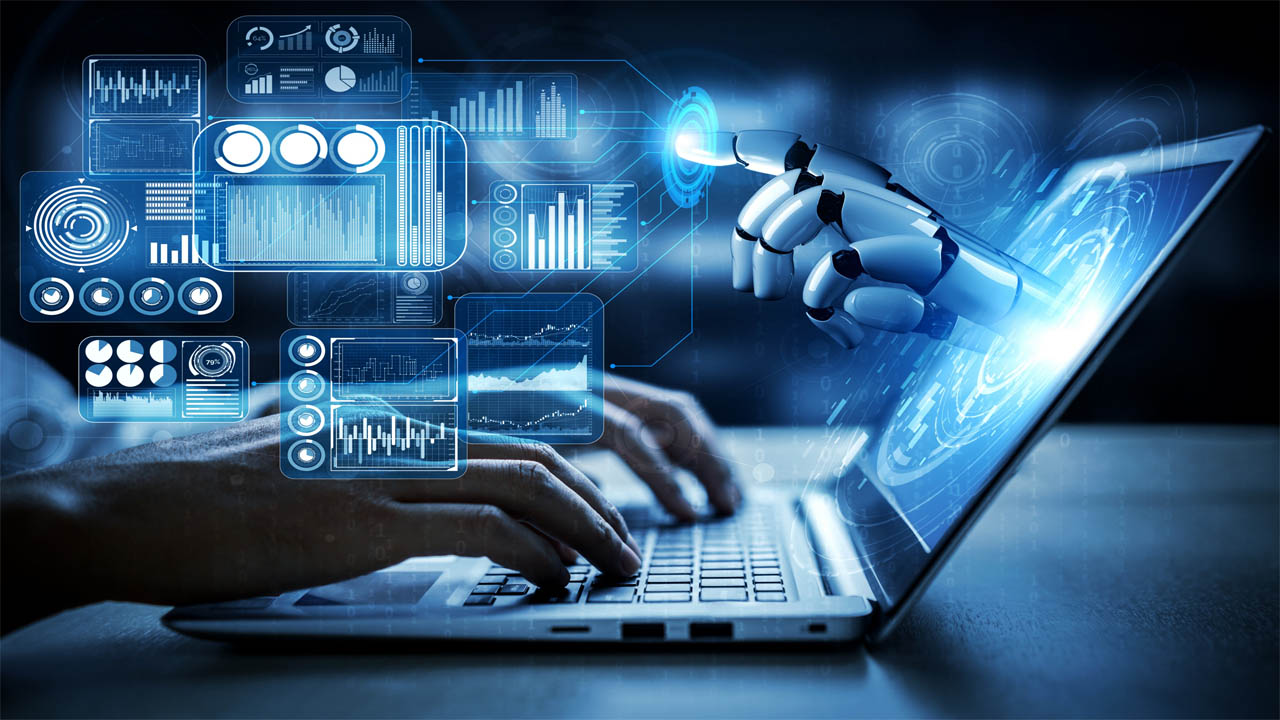
When comparing artificial intelligence vs human intelligence, remember they’re both trying to do the same things. The human brain has millions of interconnected neurons collaborating to absorb and process information. AI uses deep learning and neural networks built from layers of interconnected software nodes.
These DL models use extensive labeled data and specific neural network architectures, allowing machines to learn through experiences. Despite these advancements, human intelligence has a nuanced and adaptive nature that surpasses AI in domains like education, creativity, and robotics.
AI in education
- AI has been integrated into education to personalize learning experiences, provide instant feedback to students, and assist in administrative tasks.
- However, human educators possess emotional intelligence, empathy, and the ability to understand the unique needs of each student, aspects that AI currently cannot fully replicate.
AI in creativity
- AI has demonstrated capabilities in generating art, music, and literature. Yet, it lacks the emotional depth and originality of human creativity.
- While AI can aid the creative process by offering new perspectives or automating specific tasks, human intuition and emotion remain unparalleled in creative endeavors.
AI in robotics
- In robotics, AI has enabled advancements in autonomous navigation, object recognition, and decision-making processes.
- Nonetheless, human intelligence continues to excel in complex real-world scenarios, where adaptability and ethical decision-making are necessary.
Many things about the human mind give us an edge over our machine-based counterparts. While computers can’t recreate human emotions, they are improving their ability to understand our moods through deep learning. Subtle nonverbal cues like tone changes and frowns can help AI detect our moods.
Researchers have already tried expanding deep learning capabilities beyond mood recognition. In 2015, a team of Google engineers introduced the concept of deep dreaming. Deep dreaming involves feeding algorithms to machines, enabling them to simulate the dreaming process in human neural networks.
Researchers look to bridge the gap between human and machine intelligence as AI evolves. One promising avenue is the development of hybrid systems that combine the strengths of humans and AI. Leveraging the computational power of machines and the creativity of humans could give these hybrid systems the potential to revolutionize fields such as education, art, and robotics.
Imagine AI collaborating with human educators to create personalized learning experiences that cater to unique student needs. Consider the possibility that AI assists artists and writers in pushing creative boundaries. In some ways, we’re already on our way.
However, the goal should be to discover new ways to harness its power while preserving the invaluable qualities of human intelligence.
Final Thoughts
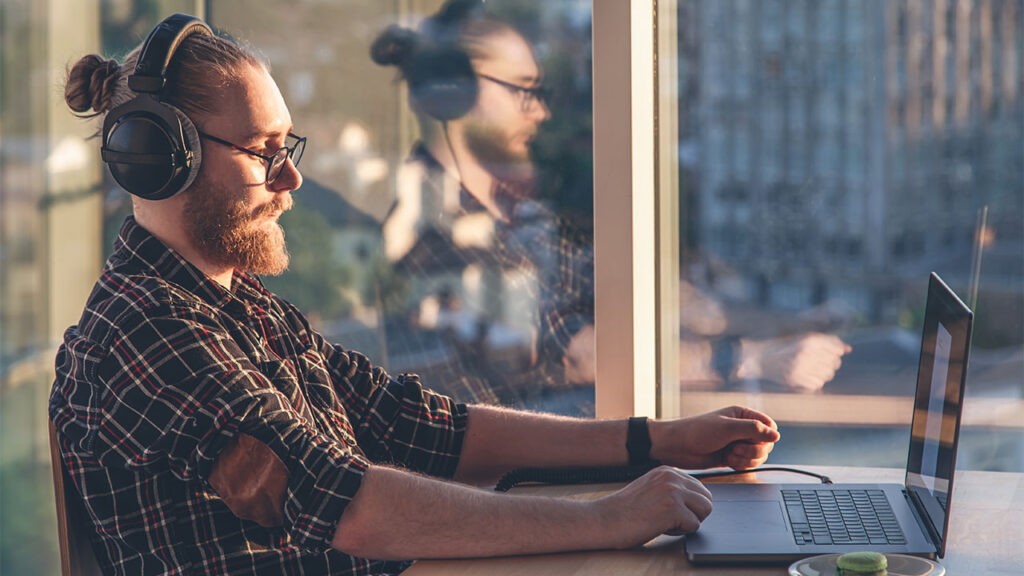
Whether spurred by DL advancements or not, our world will undoubtedly become more interconnected and automated. As AI advances, it’s crucial to consider the ethical implications of integrating it further into our lives. While AI can enhance efficiency and productivity, it shouldn’t replace human connection and empathy.
Striking a balance between the capabilities of AI and the unique qualities of human intelligence will be vital in shaping a future where technology serves and preserves humanity.